AI Ethics: Addressing Bias and Fairness in Machine Learning Models
Artificial Intelligence (AI) holds immense promise to revolutionize industries, drive innovation, and solve complex problems. However, as AI technologies become increasingly integrated into our daily lives, concerns about bias and fairness in machine learning models have come to the forefront. In this article, we explore the ethical implications of AI, the challenges posed by bias in machine learning, and strategies to promote fairness and accountability in AI systems.
Understanding Bias in Machine Learning
Bias in machine learning refers to systematic errors or inaccuracies in the output of algorithms that result from flawed assumptions, incomplete data, or societal prejudices. These biases can manifest in various forms, including racial, gender, or socioeconomic biases, and have far-reaching implications for decision-making processes in areas such as hiring, lending, and criminal justice.
One of the primary challenges in addressing bias in machine learning is the reliance on historical data, which may reflect existing societal biases and perpetuate inequalities. For example, biased training data can lead to discriminatory outcomes, such as algorithmic bias in hiring practices or predictive policing systems that unfairly target minority communities.
Promoting Fairness and Accountability in AI
Addressing bias and promoting fairness in AI requires a multi-faceted approach that involves stakeholders across various domains, including policymakers, technologists, and ethicists. Some key strategies to promote fairness and accountability in AI include:
- Diverse and Representative Data: Ensuring that training datasets are diverse, representative, and free from biases is essential to mitigating algorithmic bias. Data collection processes should be transparent, and efforts should be made to include underrepresented groups to prevent skewed outcomes.
- Algorithmic Transparency: Increasing the transparency of AI algorithms and decision-making processes can help identify and address biases. Explainable AI techniques, such as model interpretability and transparency tools, enable stakeholders to understand how algorithms arrive at their decisions and identify potential sources of bias.
- Bias Detection and Mitigation: Implementing bias detection and mitigation techniques within machine learning pipelines can help identify and mitigate biases before they manifest in AI systems. Techniques such as bias audits, fairness-aware algorithms, and adversarial testing can help detect and address biases at various stages of the development lifecycle.
- Ethical Guidelines and Standards: Developing ethical guidelines and standards for AI development and deployment can provide a framework for responsible AI governance. Initiatives such as the AI Ethics Guidelines by professional organizations and industry consortia aim to promote ethical AI practices and raise awareness about the societal impact of AI technologies.
- Continued Education and Awareness: Educating stakeholders about the ethical implications of AI and bias in machine learning is crucial to fostering a culture of responsible AI usage. Training programs, workshops, and awareness campaigns can help raise awareness about bias and promote ethical decision-making in AI development and deployment
Conclusion
As AI technologies continue to advance, addressing bias and promoting fairness in machine learning models is paramount to ensuring equitable and responsible AI deployment. By adopting ethical guidelines, promoting transparency, and implementing bias detection and mitigation strategies, we can harness the transformative potential of AI while minimizing the risks of algorithmic bias and discrimination. Together, we can work towards building AI systems that are not only technologically advanced but also ethical, fair, and accountable to all members of society.
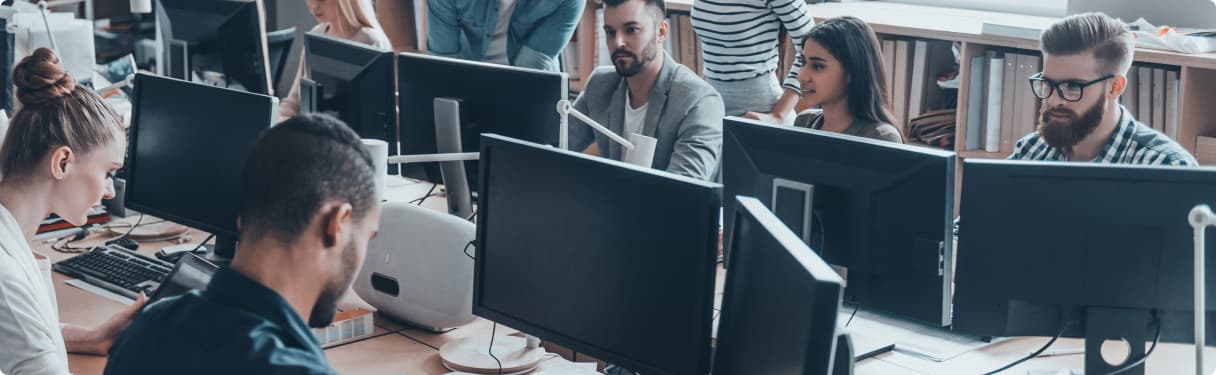